In the vast realm of technological advancements, two fields have emerged as particularly transformative: quantum computing and artificial intelligence (AI). Both have been subjects of intense research and speculation, promising to redefine the boundaries of what’s possible in the digital age. This article seeks to explore the fascinating intersection of these two domains, particularly focusing on how quantum computing is revolutionizing AI-driven data analysis.
Quantum Computing: A Brief Overview
Quantum computing, at its core, is a radical departure from the classical computing models we’ve grown accustomed to. While classical computers use bits as the smallest unit of data, which can be either 0 or 1, quantum computers use quantum bits or qubits. These qubits can exist in a state of 0, 1, or both simultaneously, thanks to a phenomenon known as superposition. This ability to process multiple possibilities at once gives quantum computers a computational advantage, especially for specific complex problems.
The Growing Importance of AI-driven Data Analysis
In today’s data-driven world, the ability to analyze vast amounts of information efficiently is paramount. From healthcare to finance, AI-driven data analysis plays a crucial role in extracting meaningful insights from raw data. Machine learning models, a subset of AI, are trained on large datasets to make predictions or decisions without being explicitly programmed. The efficiency and accuracy of these models are often limited by the computational power available. This is where the potential of quantum computing comes into play.
Setting the Stage for a Technological Synergy
Imagine a world where machine learning models can be trained in seconds instead of days, where real-time data analysis on massive datasets becomes the norm, and where AI’s predictive capabilities are exponentially enhanced. This isn’t a distant dream but a foreseeable future made possible by the convergence of quantum computing and AI.
Understanding Quantum Computing
Quantum computing represents a paradigm shift in our approach to computation, leveraging the principles of quantum mechanics to process information in ways that are fundamentally different from classical computers. At the heart of this technology are quantum bits, or qubits, which can exist in multiple states at once thanks to superposition. This capability allows quantum computers to perform complex calculations at unprecedented speeds.
Quantum Bits and Superposition
Classical computers use bits as the basic unit of information, which can be either a 0 or a 1. In contrast, qubits can be in a state of 0, 1, or both simultaneously. This phenomenon, known as superposition, enables quantum computers to process a vast number of possibilities at once, providing a significant advantage for certain types of calculations.
The Quantum Advantage
The true power of quantum computing lies in its ability to solve problems that are currently intractable for classical computers. For example, factoring large numbers, simulating quantum systems, and optimizing complex systems are areas where quantum computers have the potential to excel. This quantum advantage opens up new possibilities in various fields, from cryptography to material science.
Aspect | Classical Computing | Quantum Computing |
---|---|---|
Basic Unit of Data | Bit (0 or 1) | Qubit (0, 1, or both) |
Processing | Sequential | Parallel (for certain calculations) |
Best Suited For | General-purpose computation | Specific complex problems |
Current Development | Mature technology | Still in experimental stages |
AI-driven Data Analysis: The Current Landscape
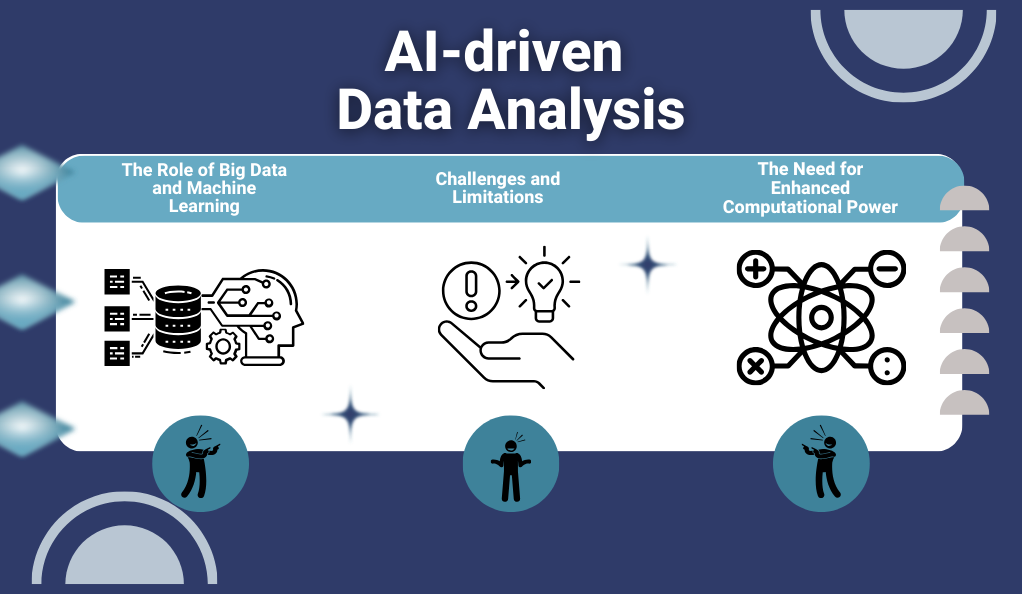
The integration of artificial intelligence in data analysis has revolutionized the way we interpret and utilize data. Machine learning models, a subset of AI, are trained on large datasets, learning patterns and making predictions or decisions without explicit programming. This shift towards data-driven decision-making is evident across various industries, from healthcare diagnosing diseases to financial institutions detecting fraudulent activities.
The Role of Big Data and Machine Learning
The surge of big data has been a significant catalyst in the evolution of AI-driven data analysis. Machine learning models thrive on data; the more data available, the more accurate and reliable the models become. Big data provides the necessary volume of information, while machine learning algorithms analyze and extract valuable insights, transforming raw data into actionable knowledge.
Challenges and Limitations
- Despite advancements, AI-driven data analysis faces challenges.
- Processing large datasets and training complex models requires immense computational power.
- Classical computers, especially with big data, have speed and efficiency limitations.
- The accuracy of machine learning models depends on data quality and algorithm choice, sometimes resulting in biased or inaccurate results.
The Need for Enhanced Computational Power
As datasets continue to grow in size and complexity, the need for enhanced computational power becomes increasingly apparent. The limitations of classical computing necessitate a new approach to data processing and analysis. This is where quantum computing enters the scene, offering a potential solution to the computational challenges faced in AI-driven data analysis.
Quantum Computing’s Impact on Machine Learning and Data Processing
Quantum computing introduces a new paradigm in data processing, providing a significant speed-up for specific calculations and algorithms. This enhancement is particularly beneficial for machine learning models, where the ability to quickly process and analyze large datasets is crucial.
Quantum Algorithms and Machine Learning
Quantum algorithms are being developed to enhance machine learning models, offering faster training times and improved performance. For example, the Quantum Approximate Optimization Algorithm (QAOA) is designed to solve optimization problems more efficiently than classical algorithms, directly benefiting machine learning models that rely on optimization.
Processing Large Datasets with Quantum Computing
The inherent parallelism of quantum computing makes it exceptionally well-suited for processing large datasets. Quantum computers can analyze multiple possibilities simultaneously, providing a substantial speed advantage over classical computers for specific data analysis tasks.
Real-world Applications and Use Cases
- Integration of quantum computing in AI-driven data analysis is in the experimental stage.
- Emerging potential use cases include optimizing supply chains and enhancing drug discovery processes.
- The combination of quantum computing and AI has the potential to transform industries.
- It can solve complex problems more efficiently than ever before.
Challenges and Considerations in Integrating Quantum Computing with AI
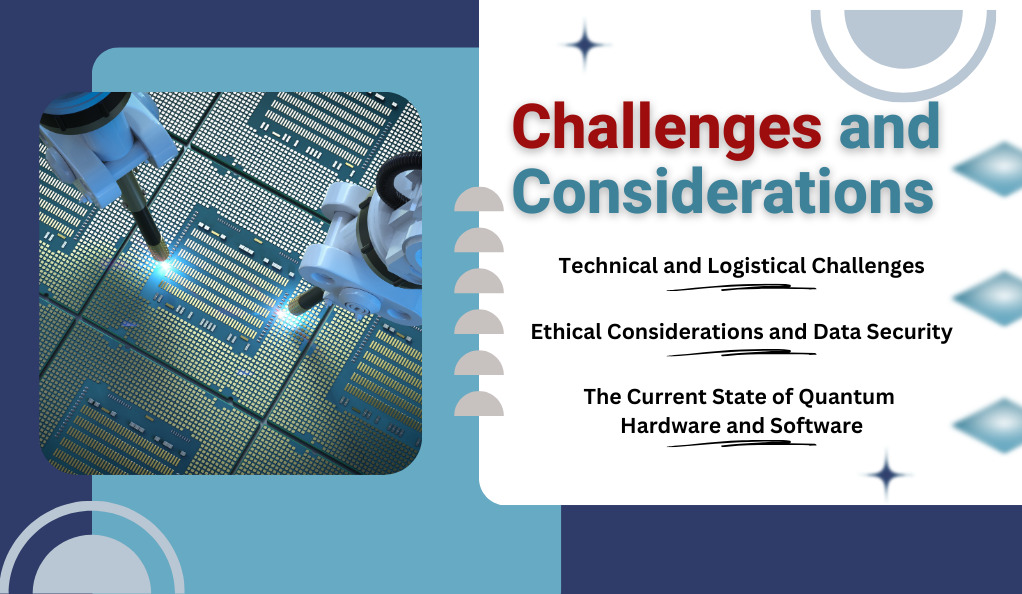
Merging quantum computing with AI-driven data analysis is a venture filled with potential, yet it is not without its hurdles. The nascent stage of quantum technology, coupled with the complexity of AI systems, presents a unique set of challenges and considerations that must be addressed to unlock the full potential of this technological synergy.
Technical and Logistical Challenges
Quantum computers, with their need for extremely low temperatures and stability, pose significant technical challenges. The hardware is delicate, and maintaining the coherence of qubits is a complex task. Additionally, the development of quantum algorithms that can outperform their classical counterparts, especially in the realm of AI and machine learning, is an ongoing area of research.
The integration of quantum computing with existing AI systems also presents logistical challenges. The infrastructure for quantum computing is vastly different from classical computing, necessitating new approaches to data storage, transmission, and processing. Ensuring compatibility and seamless integration between quantum computers and existing AI systems is a critical task that requires careful planning and execution.
Ethical Considerations and Data Security
The integration of quantum computing and AI also raises ethical considerations, particularly in terms of data privacy and security. Quantum computers have the potential to break current encryption methods, posing a significant threat to data security. As such, the development of quantum-resistant encryption methods is crucial.
The enhanced capabilities of AI systems, when powered by quantum computing, also necessitate a reevaluation of ethical guidelines. The potential for bias in AI models, the transparency of decision-making processes, and the impact on employment are all areas that require careful consideration and responsible management.
The Current State of Quantum Hardware and Software
The field of quantum computing is still in its early stages, and the availability of quantum hardware and software is limited. The development of quantum computers that are stable, reliable, and accessible is a crucial step in bringing the benefits of quantum computing to AI-driven data analysis.
Future Prospects: Preparing for a Quantum-Powered AI Future
As we navigate the challenges and considerations of integrating quantum computing with AI, the future prospects of this technological synergy are vast. The potential for breakthroughs and innovations is significant, promising to transform industries and enhance our capabilities in data analysis and decision-making.
Predictions and Innovations on the Horizon
The continued development of quantum computers is expected to lead to significant advancements in AI-driven data analysis. Quantum-enhanced machine learning models, capable of processing large datasets with unprecedented speed and efficiency, are on the horizon.
The integration of quantum computing and AI is also expected to lead to innovations in optimization, simulation, and problem-solving, opening new frontiers in research and application. From healthcare and finance to logistics and manufacturing, the impact of this technological convergence is expected to be far-reaching.
Preparing for the Quantum Revolution
As we stand on the cusp of a quantum revolution, it is crucial to prepare for the changes and opportunities that lie ahead. This preparation involves investing in education and training, developing new tools and frameworks, and fostering a culture of innovation and collaboration.
Conclusion: Embracing the Quantum-AI Synergy
The convergence of quantum computing and AI in data analysis marks a transformative moment in technology, promising faster and more efficient problem-solving. While challenges exist, the potential benefits span various industries and aspects of society.
This ongoing journey of innovation is still in its early stages, with much to explore. Embracing a proactive approach, staying informed, and investing in skills and technology will be key to unlocking the full potential of this powerful synergy between quantum computing and AI.